Have you ever wondered 'what do Data Scientists do with data'?
Well, we've got the answer for you.
In this blog post we ask (and answer) the questions what is data?, why is data so important?, what can you do with data? and what do Data Scientists do with data?
Better still, we give you our Top 12 Tips on what you can do with your data...
More...
What Is data?
Information is all around us. It is in everything we see, hear, smell, touch and taste. It can be found in the largest event, like the formation of a new galaxy, and in the smallest, such as the spin-state of an electron (you can tell I'm a physicist, can't you?)
Simply put, data is a collection of facts and information that we have gathered and translated into a form that is convenient to process.
It can be numbers, words, measurements, observations or even just descriptions of things.
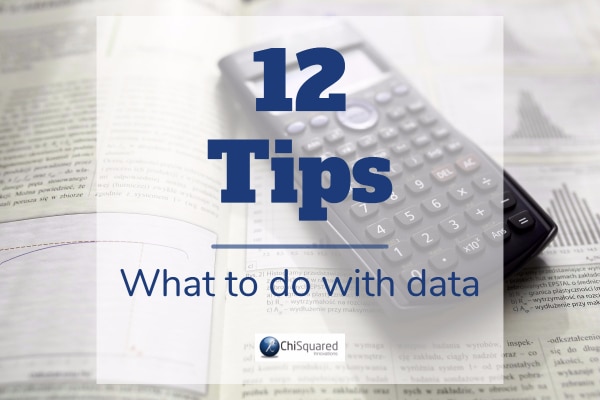
Why Do Data Scientists Collect Data?
Information on its own can be interesting, but it is not really very useful. We need to collect data so we can find out 'what the world is like'.
We might observe things like:
The questions to ask might be:
In each of these cases we need to gather the information, observe it, measure it, count it and categorise it so that we can begin to understand the 'story' behind the information.
What do Data Scientists Do With Data?
To answer the question of what do Data Scientists do with data, we typically collect data to answer one of 2 questions:
The infographic below might help to explain the difference between these questions:
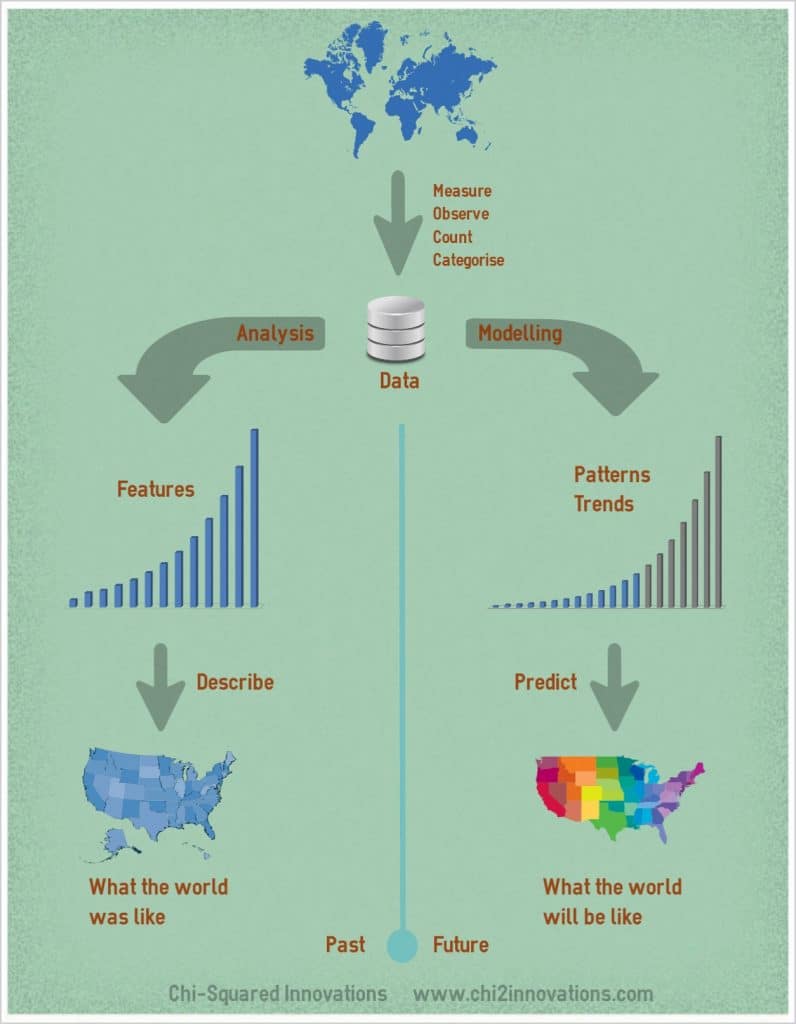
Infographic: We analyse data to find features, patterns and trends that enable us to describe what the world is like and predict what it will be like in the future
We might want to analyse the data to find features that describe to us what the world was like at the time the data was collected.
There is a whole branch of statistics dedicated to finding these features, and typically we use descriptive methods to measure things such as:
What these can't do is tell you the future.
For this we need to create models that can spot patterns and trends that allow us to predict what the world will be like in the future.
There are many different ways of producing predictions and forecasts from data, but they can be broadly grouped into 2 techniques:
I'll talk about these in greater detail in future posts.
What Do Data Scientists Do About Data Accuracy?
Ultimately, data is information that can tell us how the world works, and this is important if we want to be able to predict the future with any degree of accuracy.
If we want accurate predictions, then we need accurate data, so it is of the utmost importance that we take care when we observe and measure.
As a statistical consultant I have lost count of the number of times that I have had to tell a researcher that his/her data is not fit for purpose and if they want their questions answered correctly and accurately they need to start again.
For a 3 year PhD student with just a month to go before submitting their thesis, this is not what they want to hear - and not what I want to tell them!
So how do we know when our data is not up to scratch?
There is a whole branch of statistics dedicated to answering this question (which I'm not going to go into here), but one of the questions we can ask is:
Are our data biased?
EXAMPLE:
An example of how to detect bias in data is to check the remainder (the right-hand side of the decimal point) of continuous measurements.
Say that we are measuring the heights of 10 year old children to 1 decimal place.
We'll have measurement such as:
Now leave off everything to the left of the decimal point and we have:
Count up all the .1s, the .2s, .3s, etc., and plot the counts against the remainder.
We expect to see approximately the same number of children in each of the deciles (the .1s, .2s, .3s and so on) so the plot should be square-ish (below left):
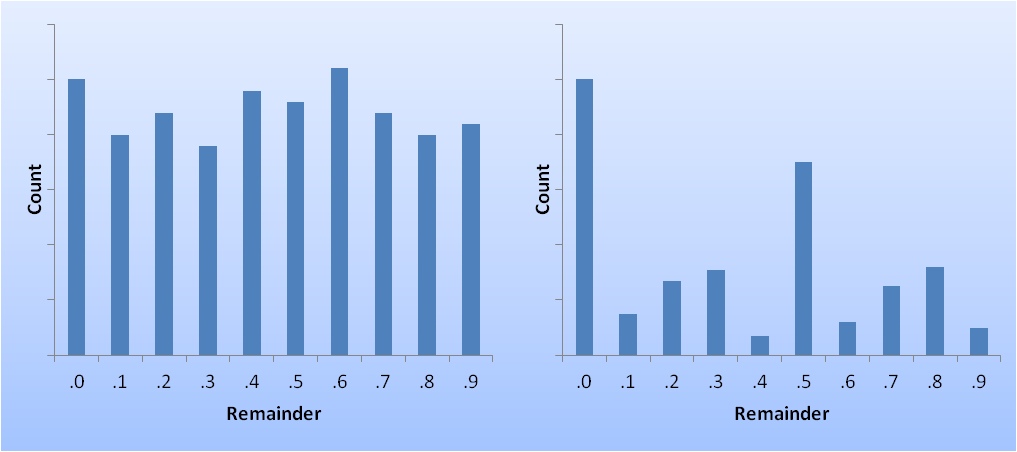
Example of how to detect bias in the data
If we see the graph on the right, we'll know that something has gone wrong with our measuring procedures.
Most likely the person/people doing the measuring have rounded to the nearest .0 or .5 for some (but not all) of the measurements, and this has inevitably introduced bias into the data.
Is this a problem?
Well, it might be, but it all depends on what questions you are asking and how accurate you need the answers to be.
Only you can answer that question, and it would be a really good idea to discuss this with your local friendly statistician before you begin collecting your data rather than just hours before an important deadline!
I wish I had a pound for every time I'd told that to someone...
Lessons Learnt...
So what have we learnt from this? As promised, here are our Top 12 Tips about what to do with data:
Tip #1: We can use data to describe what the world is like
Tip #2: We can use data to predict what the world will be like
Tips #3-12: The accuracy of our data is 10 times more important than what we plan to do with it!
So the next time you collect data, remember GIGO:
Garbage In, Garbage Out...
And maybe, just maybe, your statistician won't ruin your day by telling you to scrap your data and start again.
So if you've ever asked yourself the question 'what do Data Scientists do with data?', well now you know...